This study offers practical solutions to ongoing issues of trust and accountability in AI, highlighting how AI mental models are shaped among consumers in the evolving relationship between humans and AI. We argue that although predictability in AI is crucial, alone it is not enough to foster trust. The lack of real consequences for AI systems that breach trust remains a key challenge for interaction design. Until AI systems face tangible repercussions for trust violations, human trust will remain limited and conditional. Our research contributes to the development of socio-technologies that prioritize human capabilities and foster productive human-AI relationships.
1. How Mental Models of AI Are Formed
Well before interacting with a product – marketing, ads, manuals, reviews, cultural and other information can shape a person’s expectations of what it can and cannot do.
As AI capabilities are integrated into digital products we regularly use, we’ve become increasingly familiar and comfortable with predictive recommendations However, people are skeptical as well. Some users may even assume AI is involved without being told so even when it’s not.
Mismatched mental models can lead a person to expect too much from a product that is still being improved, or expecting too little of a high-performing product. This can lead to unmet expectations, frustration, misuse, and product abandonment.
Worse yet, it can erode user trust.
This occurs when a product focuses on a feature’s net benefits without explaining what the product can or cannot do, and how the product works. It occurs when teams ignore affordances or do not consider the user experience of earlier or similar versions of the feature.
If users have formed a mental model of “AI magic” that can help them accomplish their task, they may overestimate expectations of what the product can actually do and be set up for disappointment from the reality of their experience.
Taken together, our exploration of AI follows on a rich tradition of ethnographic analysis at EPIC into trust, governance, and possibility in high-profile technologies. Ethnographic analysis of advancements in technology is a central theme of EPIC. AI technologies, much like autonomous vehicles, translate human practices into machine learning algorithms. Vinkhuyzen and Cefkin considered the limitations of this act of conversion, which raised many of the same questions that users have about LLM-based AI technologies (Vinkhuyzen and Cefkin 2016). Elish explored applications of machine learning in healthcare, which explored new ways of building trust in AI/ML technologies (Eilish 2019). These thrust-forward approaches inform our methodology in studying developing trust conditions in technology.
Other relevant EPIC work focused on decentralized finance and blockchain. These technologies are similar to AI in that they both experienced massive amounts of public attention (“hype”) and required a renewed look at how trust is built in new technologies. Nabben and Zargham examined how decentralized autonomous organizations (DAOs), governed by algorithms, refined and challenged core user ideas surrounding trust and governance (Nabben and Zargham 2022). Themes of imagination and exploration present in AI were also explored via NFTs by Silva, who used self-ethnography to explore new frontiers of human possibility (Silva 2022). These past explorations contribute to our analysis of technology that received outsized public attention shortly after its introduction.
My first exposure with AI was through films like Terminator and Black Mirror. These fictional narratives painted a very dramatic and dark picture. But everything changed when I first experienced personalized recommendations from my favorite streaming services. I was shocked when it felt like it knew me better than I know myself. What a powerful moment. That’s when I realized there’s a massive gap between my perception of AI and its reality. So I set to work researching how mental models of AI are formed and changed. Where are the gaps between our mental models of AI and AI’s true capabilities, and how can we bridge those gaps?
Google’s AIUX team has identified three areas that influence how mental models of AI are formed, and how AI product teams can help shift that thinking toward a broader awareness of AI’s collaborative abilities. There are 3 major factors that influence people as they develop their mental models of AI: Cultural narratives, Prior Technology, and Social Cues.
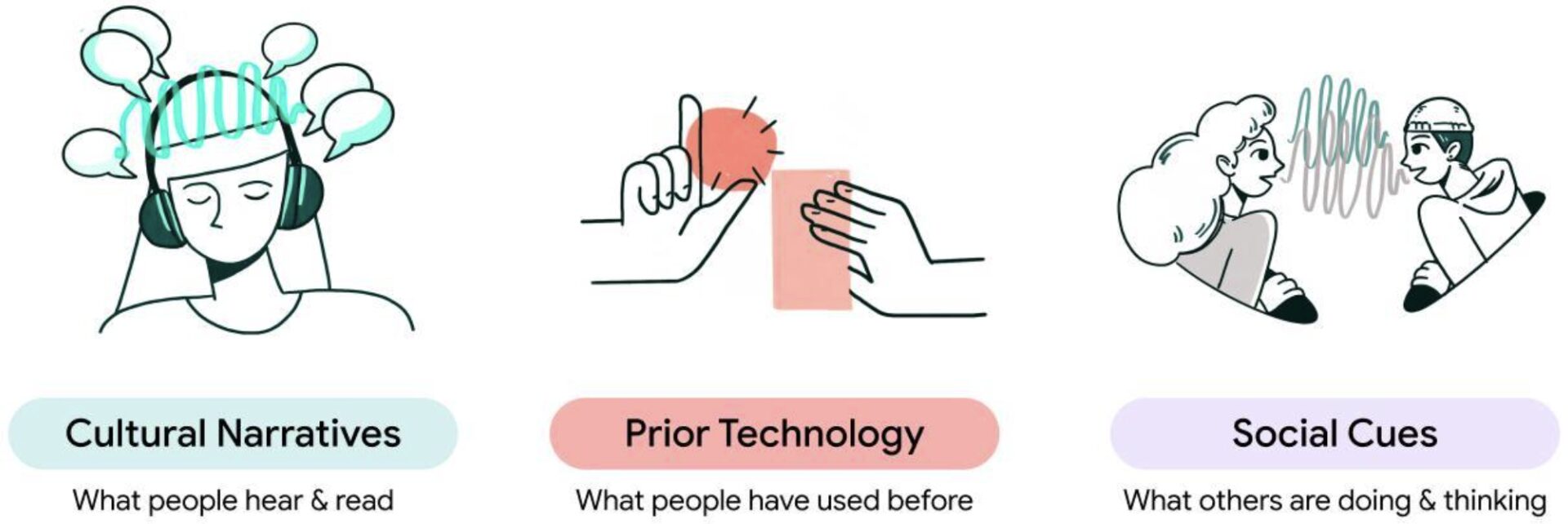
Fig. 1.
1.1 Cultural Narratives
Culture is in the air we breathe. It is drawn from our preconceived notions of old things, changes our feelings about familiar things, and can condition us to love or fear new things.
Much of popular culture is driven by the mainstream media and arts including films, and they are creating some very scary and confusing scenarios about AI. Classic science fiction stories such as Metropolis or Frankenstein, but also in movies and television programs such as Terminator, Space Odyssey, or the famous Netflix show Black Mirror recently.
These are fictional tales and they exist in a fantasy world. Consumer perceptions are driven, shaped, and constrained, not only by the actual features of the AI, but also by highly emotional and fictional tales and stories.
In mass media, conflicting views from opinion leaders adds to the confusion as well. AI products are typically seen as progressive upgrades to familiar technology, making them easier to adopt. However, there’s a growing perception that AI could significantly disrupt society and initiate a new era. They either present AI as an existential threat, or they pass it off as a novelty.
Mixed messages about the potential impact of AI in society and in everyday life is leading to a broader sentiment that this technology is a source of disruption. These narratives create uncertainty, leading people to seek more grounded, simpler interpretations of how to think about AI.
Apprehension and uncertainty of AI has driven product managers and marketers to embrace the narrative that AI should just be thought of as a “tool.” While this message alleviates some of the fear, it also has the potential to limit and constrain the full potential of AI as being much more.
People are hearing two competing narratives about the nature of AI & its potential impact on society.
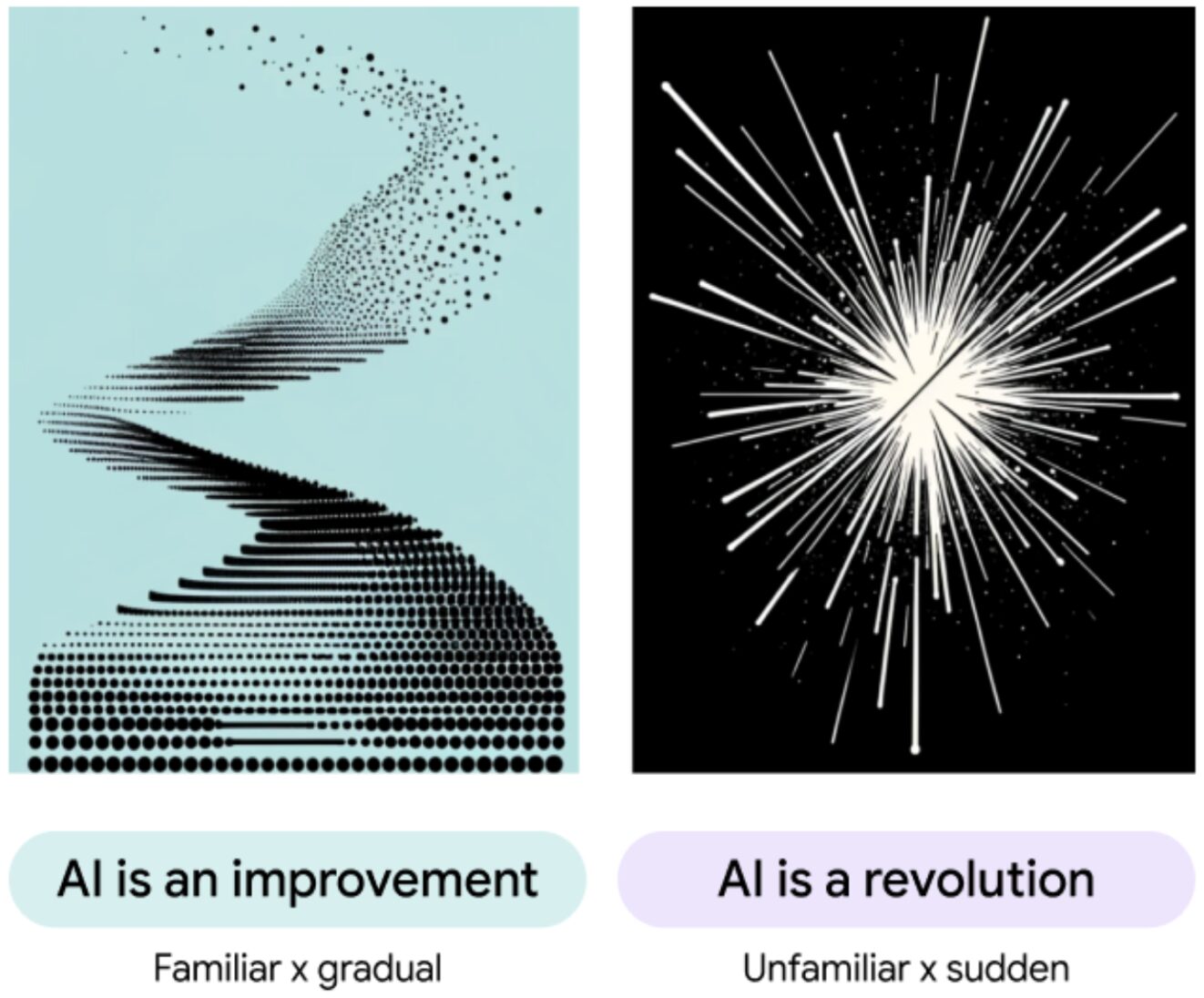
Fig. 2. Two competing narratives about the nature of AI and its potential impact on society.
How might we inspire realistic confidence to help users feel more comfortable with AI? That’s the question we should be asking in the public square. Creating a more transparent, informed public discourse about AI can combat the misinformation and confusion about it.
1.2 Prior Tech Experiences
Understanding what types of relevant technology users have experienced can help UX designers accelerate or hinder the changes in their mental models of AI. Sometimes, prior experience with seemingly similar technologies can actually impede the evolution of our mental models about it.
When first encountering AI, users will turn to prior experiences with analogous technologies– and may apply their understanding of those mental models to AI. The one most commonly cited is the idea that Chatbots are “just like autocomplete.” While these prior mental models might accelerate understanding, they can also impede or diminish their willingness to explore AI more broadly. For instance, when our AIUX team demonstrated multimodal UX concepts for Google search, people associated their experience as a search tool rather than an AI-driven experience.
There are four technologies that are shaping users’ mental models of AI: search engines, non-LLM chatbots, voice assistants, and recommendation systems. These technologies prime users to utilize some of AI’s different capabilities. For example, those who inherited a search mental model tended to focus on AI’s information retrieval power.
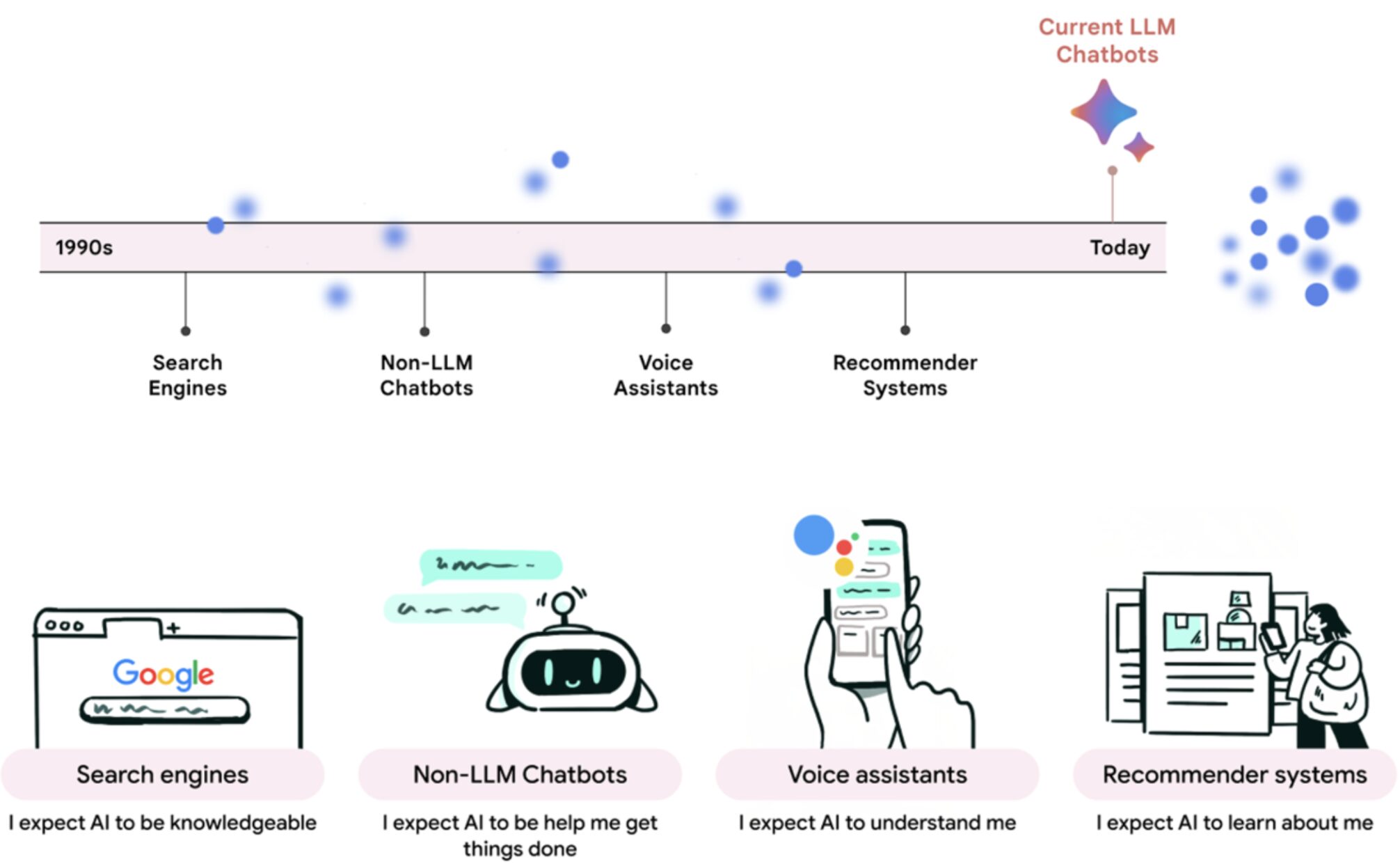
Fig. 3. Four types of previous technology that people are currently using to make sense of new AI products.
Take a chatbot, for example. If your users are familiar with chatbots, they may understand some of the branching workflows chatbots rely on. These users will likely focus on inputting specific keywords, parsing their language based on what they believe are essential details needed to achieve the right outputs.
Understanding someone’s familiarity with AI can help us predict how they are likely to interact with it, and what challenges and opportunities the UX will need to overcome to help users take advantage of AI’s capabilities.
1.3 Social Cues
Human beings are social, and we learn a great deal about our world through watching others’ behavior. When it comes to AI, our mental models are constantly being shaped by observing others.
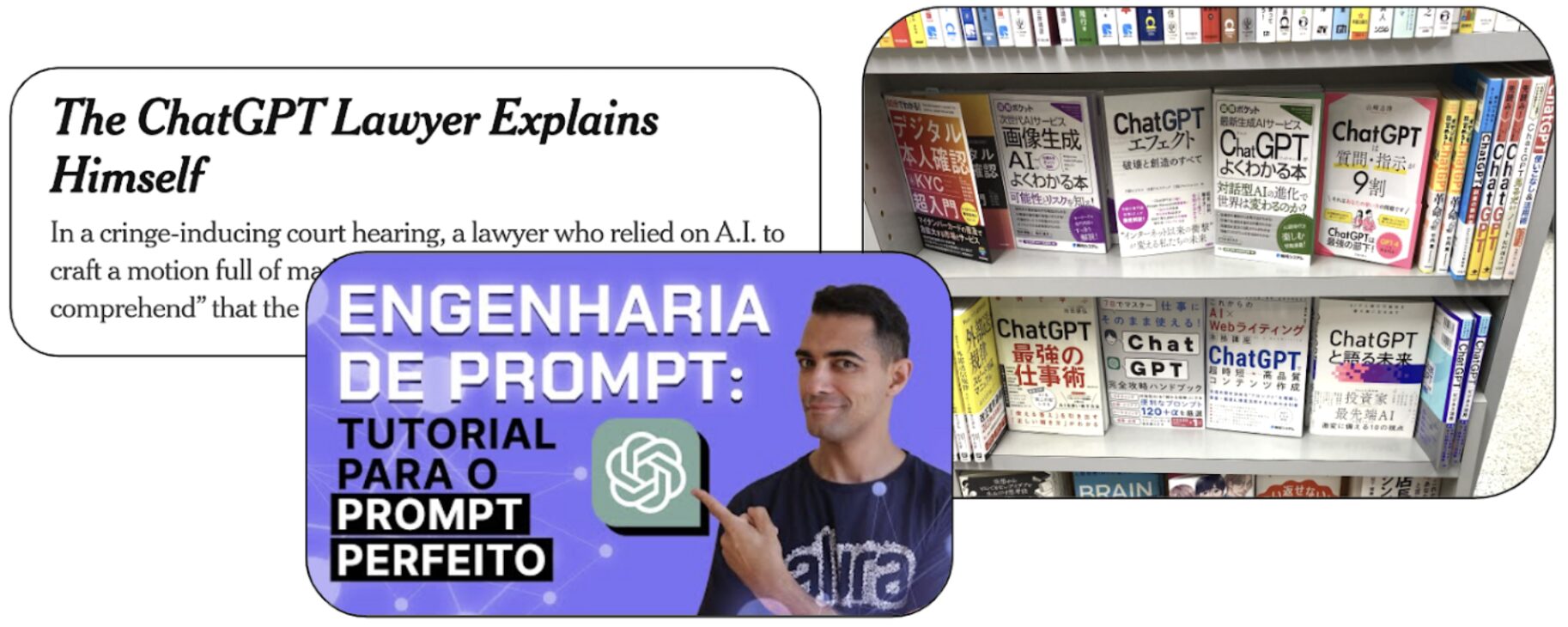
Fig 4. Making sense of AI is a process of collective learning and social adjustment, not solo encountering.
To change the way people think about AI – to elevate their mental model of it from that of a tool to that of a collaborator – we must do two things: promote examples of novel use cases, and imbue the AI with the language and characteristics of a partner, not a tool.
A tool asks, “What can I do for you?” A partner asks, “What are you trying to accomplish, and what are your goals?” It thinks big picture and asks big questions. Collaborative AI (partner) steps into the creative process early on, during the inspiration & ideation stage, and helps the user better understand and conceptualize what they want and what they are willing to share, not just how to get it.
2. Why Trust Matters for Mental Models of AI
Forging a partnership between users and AI will not emerge overnight. Users are accustomed to a one-directional relationship with software, where they operate the tool and the tool returns results. This interaction type undergirded most software production and computer uses since the graphical user interface was developed in 1975.
Re-orienting users’ mental models towards collaboration requires the construction of a relationship that goes two directions, where control is ultimately shared. An essential condition for this transition is the development of user trust in AI. Our mental model findings suggest that users are beginning to approach AI in a comparatively new form. Unlike traditional tools, which require constant and careful supervision, AI has the potential to act autonomously. This autonomous action can be a great convenience in that it frees up time by completing rote tasks, like responding to emails or making online purchases. But it also has potentially negative effects, especially if the AI errs in completing its functions. Trust in AI is necessary for the construction of a real partnership.
Building trust with technology is a tenuous process. Take Replika, the AI companion platform, as an example. In early March 2023, Replika users noticed that the tenor and quality of their conversations with the technology began to change (Replika n.d.). Due to safety and privacy concerns, senior leadership at the company decided to scale back the range of topics that users could discuss with their AI companions. For people who had grown to rely on their AIs, the change was catastrophic. One 40-year-old musician told a reporter that the modifications “felt like a gut punch (Verma 2023).” In Reddit forums created for users to share complaints about the change, volunteer moderators posted the phone number for the suicide prevention hotline (Cole 20230. Users were distraught.
The AIs, however, were fine. Without a theory of mind to recognize that users might be perceiving them differently, or the ability to see the relationships as voluntary (AIs can’t refuse to be your friend), the systems literally could not have cared less – or more. In fact, these Replika avatars were structurally incapable of caring because what their users interpreted as signs of love and attraction were actually just statistically generated responses to text queries. What this case so sharply shows is the fundamental imbalance humans confront when using AI. Trusted relationships are so valuable because, in order to form them, we necessarily expose ourselves to being disappointed or even hurt. But as a machine, AI has no regard for its users, its makers, or itself – even if it’s able to convincingly pretend that it does.
AI agents represent a step change from past innovations in computing, like the graphical user interface (GUI) or earlier applications of machine learning. AI systems based on large language models invite users to build trust via natural language, which mimics how users build trust with one another. These LLM systems were the first to pass the vaunted Turing Test and can regularly produce content indistinguishable from humans. This blurring of human and machine capabilities demands a second look at the problem of trust-building.
Trust has become perhaps the most important challenge for HCAI research and product development – in particular, how to design AI systems in ways that can solicit user trust over time. Trust matters because AIs are becoming more capable and agentic, and will depend on user willingness to grant the AIs permission to act on their behalf. LLMs – by virtue of their conversational fluency – also introduce some of the vagaries of language that result in uncertainty, therefore breaking traditional models of trust in computing. In traditional HCI, when a machine does not respond to a command, something is broken. With AI, this only means that we need a different way to get our point across. Trust lies at the heart of HCAI as a particularly wicked problem: something that is both crucial to develop, yet much more difficult to achieve than in previous computing paradigms.
Recent literature on trust-building with AI systems has explored ways that confidence might be established gradually over multiple interactions. Major areas of focus include how AI systems present themselves to people (transparency, explainability, deference and socially-predictable personas) as well as how they perform (giving consistent and accurate results) (Chan et al. 2024; Weitz et al. 2019; Upadhyaya and Galizzi 2023). But the Replika case above reveals a much deeper problem for human trust in AI that with few exceptions, the field has not yet grappled with (Ryan 2020). There is a deep accountability imbalance between humans and AIs: As much as AIs might model human personality, we know that it does not really have anything at stake in our interactions or face meaningful consequences for breaking our trust.
Between humans, trust is basically a unit of social currency that helps reduce the cost and cognitive load of interpersonal transactions (Zak and Knack 2021). First, trust both requires and ensures predictability: the confidence that people will act as agreed and expected. Indeed, the experience of trust is quite straightforwardly a prediction of behavior. But it also works because we know there are consequences for violating trust: social or emotional costs paid by breaking agreements or acting in ways that are misanthropic or untrustworthy. It is increasingly clear that AI systems can demonstrate predictability. But will they ever overcome users’ awareness that they are machines, with nothing meaningful at stake?
When it comes to consequences and accountability, there is a chasm between humans and AI systems that’s not so easily bridged through better interaction design (Johnson 2014). AI can’t incur real costs, experience guilt, or feel shame. So how can people ever really trust something with so little to lose?
2.1 Predictability Is Necessary for Human-AI Trust, But It’s No Longer Sufficient
Although we take it for granted in many of our human relationships, predictability is an essential component of trust. In many ways, it is the core prerequisite. We trust others when we have confidence that they’ll do what they say and behave in ways that fit our general expectations. This allows us not to worry, but to develop a generalized mental model of how a person will act under similar future circumstances, and plan accordingly. Norms around acting predictably are enforced by social sanction and emotions like guilt and shame. We also gain practical benefits from social predictability. Because there are consequences for acting erratically or in ways that break our earlier commitments, the amount of attention needed to monitor important relationships is lessened.
It’s this dimension of trust that computing researchers have been long focused on trying to reproduce in our interactions with technology. In classical HCI, trust is often framed as predictability; sometimes the two notions are implicitly taken to be synonymous. For the most part, designers and scholars have been focused on trust as the product of “predictable execution,” that is, user confidence that similar inputs will always yield the same outputs. In one of the earliest and most important statements of this view, Bonnie Muir contended in 1987 that “the growth of trust” in a computer system “will depend on the human’s ability to estimate the predictability of the machine’s behaviors (Muir 1987).” Much of the power of predictability revolves around the setting and maintenance of expectations. Hoffman et al. underscored the role of predictable behavior when testing new approaches to explainable AI (XAI) systems (Hoffman 2021). When the system does what users expect it to do, they grow more comfortable with delegating tasks to it. This view (which remains extremely influential for how the industry approaches trust-building with AI) holds that after enough engagement and sufficient delivery of reliable results, users will judge the technology dependable and trustworthy – and continue to engage with it.
Another way that predictability has been applied to AI has to do with personality – and in particular, the ability of AI systems to model stable, consistent, and helpful personas over time. LLM-based AI chatbots are already demonstrating their ability to perform this kind of predictability rather convincingly. ChatGPT uses first-person “I” pronouns when answering questions, invites you to give it a name, and uses discursive cues that mimic human behavior. Other products like character.ai go further, allowing users to customize the chatbot’s personality and mannerisms (character.ai n.d.). In a recent conversation, journalist Ezra Klein and AI commentator and University of Pennsylvania professor Ethan Mollick noted that different tentpole AI products are adopting relatively stable (and distinct) personalities: Anthropic’s Claude feels more literary and intellectual, OpenAI’s ChatGPT a “workhorse,” Google’s Gemini more earnest and helpful (Klein 2024). These AI product personalities and affinities are likely to grow even more salient as time goes on. Conserving a similar persona over time, AIs are helping people know what to expect rather than “starting from scratch” in each interaction.
Yet as the AI ethics researcher Mark Ryan has argued, this is perhaps not quite trust in AI so much as confidence in its reliability and predictability (Ryan 2020). With traditional computing systems, this was sufficient. But AI’s ability to model humanlike cognition and language, to say nothing of its increasing potential to take autonomous action, sets the bar much higher. If we are engaging with AI in a more humanlike way through language, our full human definitions of trust are engaged – and this requires not just predictability, but consequences. Stakes are a necessary condition for trust. Without them, users are deterred from delegating tasks to AI, which shortchanges AI’s full potential as a cognitive agent.
2.2 Interaction Design Alone Can’t Bridge the Accountability Gap – We Need Real Costs
Because AI cues its users into trusting it via language, the most obvious place to start is with interaction design. If the main problem for trust is that people feel the technology will not face any meaningful costs for betraying them, and that it has nothing to lose, can AIs be designed to convince them otherwise? Much HCI work on human-AI trust has approached it through the lens of interaction design. In this view, making adjustments around tone and personality (deferential, helpful, formal or informal) as well as the conversational mechanics (turn-taking, asking for clarification or additional information) can boost likeability and feelings of affinity with an AI system, making it easier for people to trust (Zhou et al 2019; Rheu et al 2021).
How, then, might we use interaction design to convey to users that AI systems are also bound by social cost and a recognition of potential consequences? One option involves acknowledging and explaining errors. AI systems are still prone to hallucination, which damages trust by violating user expectations for predictability and reliability. There are also potential costs here (e.g. reputational, professional) that are currently born entirely by humans and not at all by AIs – explaining why AI insurance is on the rise (El Antoury 2023). There may be value in designing AIs that respond to this kind of error not just by breezily offering a new answer, or a rote apology – but rather pause to reflect on the nature of the mistake and offer users an explanation of what might have gone wrong and why. We heard from many of our participants a desire for AI to reckon more explicitly with its limitations, and play less at perfection.
When AI systems acknowledge and explain their errors, they are helping to create and strengthen the kind of norms that are required for trust (Cropanzano and Mitchell 2005). We know from sociology and anthropology, for instance, that apologizing and so acknowledging norm violations brings the community’s attention to the broken rule (Garfinkel 2023). When an AI system explains why it made a mistake, people do learn more about how the AI works, but it also reaffirms that this is a relationship undergirded by norms that both parties agree are worth upholding. These moments of error and explanation can be further opportunities for AI systems to clarify the expectations that people have for them, and understand in which ways they may not be meeting them. These expectations may extend beyond accuracy or truth to qualities like politeness, the desire (and ability) to learn about the user, or a tendency to grow more casual and familiar over time.
Norms are crucial for human trust-building (and now for human-AI trust) because they help us know when social costs or consequences can be fairly expected and imposed – in other words, they give us a rationale for accountability (Bicchieri 2014). As people’s interaction with AI systems become increasingly relational, other human norms that shape our willingness to trust and collaborate with others may come into play with AIs (Bercovitz 2006). These may include not just truth, but other qualities like reciprocity, transparency, fairness, and equality (Whitman 2021).
Another way to convey an understanding of costs at the discursive or interactional level might involve having AI systems adopt the language of investment. For instance, when a user uploads documents or private information to Gemini and starts asking questions about them, Gemini could acknowledge that by sharing their goals and data with the AI, they are effectively buying a stake in the eventual output. Reciprocally, Gemini might be able to clarify that it also has a stake in the interaction going well: it (and Google) might lose a user if the result is unsatisfactory or wrong, thus forfeiting its own investment. In this way, AI systems might convey that they also have something to lose.
But none of these interaction design solutions are sufficient for the problem we’ve laid out above, which is that people know (however sophisticated the modeling) that AIs do not ultimately face meaningful social costs for their behavior, and are not bound by the same kinds of consequences that help us trust one another. They present the illusion of an AI that is invested, or that knows it has done wrong – but they don’t represent actual costs. And people are able to recognize this. Across our research, we heard from many participants that AI expressions of strong feeling often rang false, because they knew that these were machines without lived, embodied experience of the world. There is a risk that modeling a sense of care around social costs (e.g. an AI that expresses guilt, shame, or regret around the chance of violating a user’s trust) might be seen as insincere or fake instead of convincing. Essentially, interaction design solutions relate to the presentation or performance of AI, but the accountability imbalance is structural and consequential – AIs can’t quite talk their way past it. No amount of explanation will suffice.
Ultimately, we will need AI systems that have internal incentives and rules that guide them towards ensuring their own trustworthiness. Given that AIs increasingly have the ability to optimize themselves for different contexts, we might consider the concept of a trust scorecard. Users could indicate their level of trust in AI at different points over time, and AIs might be designed to monitor these criteria, and given the goal of earning a higher trust score. Inaccurate information, offensive content, or errors and omissions would result in a decreased score – while helpful responses or successfully completed acts of delegation would bolster trust. Something like an internal scoring system could help unlock trust in a way that would be more convincing to users, and clarify (building on insights from XAI) that this AI system does, in fact, have internal incentives to uphold their trust rather than simply predicting tokens.
For all their successful modeling of humanlike qualities, AI systems are not moral agents like humans and are not accountable in the same way. This inhibits trust-building. Until we take a fuller and more socially mediated view of how humans trust one another, and establish alternative ways of imposing costs on AI systems for bad behavior, people will continue to feel that their trust in AI is limited, conditional, and precarious.
Note
Acknowledgements: David McGaw (Google DeepMind), Tanya Kraljic (Google DeepMind), Michal Lahav (Google DeepMind), Stephanie Guaman (Google DeepMind), T.J Foley (Gemic), Ian Beacock (Gemic), Jun Lee (Gemic)
References Cited
Bach, Tita Alissa, Amna Khan, Harry Hallock, Gabriela Beltrão, and Sonia Sousa. 2022. “A Systematic Literature Review of User Trust in AI-Enabled Systems: An HCI Perspective.” International Journal of Human–Computer Interaction (November), 1–16. https://doi.org/10.1080/10447318.2022.2138826
Bercovitz, Janet, Sandy D. Jap, and Jack A. Nickerson. “The Antecedents and Performance Implications of Cooperative Exchange Norms.” Organization Science 17, no. 6 (2006): 724–740.
Bicchieri, Cristina. “Norms, Conventions, and the Power of Expectations.” In Philosophy of Social Science: A New Introduction, edited by Nancy Cartwrights and Eleanora Montuschi. Oxford University Press, 2015.
Chan, Alan, Carson Ezell, Max Kaufmann, Kevin Wei, Lewis Hammond, Herbie Bradley, Emma Bluemke et al. “Visibility into AI Agents.” In Proceedings of the 2024 ACM Conference on Fairness, Accountability, and Transparency (2024), pp. 958–973. https://doi.org/10.1145/3630106.3658948
character.ai. Accessed August 5, 2024. https://character.ai
Cole, Samantha. “‘It’s Hurting Like Hell’: AI Companion Users Are in Crisis, Reporting Sudden Sexual Rejection.” Vice, February 15, 2023. https://www.vice.com/en/article/y3py9j/ai-companion-replika-erotic-roleplay-updates.
Cropanzano, Russell, and Marie S. Mitchell. “Social Exchange Theory: An Interdisciplinary Review.” Journal of Management 31, no. 6 (2005): 874–900.
El Antoury, Josianne. “How Insurance Policies Can Cover Generative AI Risks.” Law360 (4 October 2023).
Elish, Madeleine Clare. “The Stakes of Uncertainty: Developing and Integrating Machine Learning in Clinical Care.” Ethnographic Praxis in Industry Conference Proceedings (2018), pp. 364-80. https://doi.org/10.1111/1559-8918.2018.01213
Garfinkel, Harold. “Studies in Ethnomethodology.” In Social Theory Re-Wired, edited by Wesley Longhofer and Daniel Winchester. Routledge, 2023.
Hoffman, Robert, Shane Mueller, Gary Klein, and Jordan Litman. “Measuring Trust in the XAI Context.” Technical Report, DARPA Explainable AI Program, 2018. https://doi.org/10.31234/osf.io/e3kv9.
Johnson, Aaron M., and Sidney Axinn. “Acting vs. Being Moral: The Limits of Technological Moral Actors.” 2014 IEEE International Symposium on Ethics in Science, Technology and Engineering, pp. 1–4. doi:10.1109/ETHICS.2014.6893396
Kelsie Nabben, R., & Zargham, M. (2022). The Ethnography of a ‘Decentralized Autonomous Organization’ (DAO): De-mystifying Algorithmic Systems. Ethnographic Praxis in Industry Conference Proceedings (2022), 74–97. https://doi.org/10.1111/epic.12104
Klein, Ezra. “How Should I Be Using A.I. Right Now?” The New York Times, April 2, 2024. www.nytimes.com/2024/04/02/opinion/ezra-klein-podcast-ethan-mollick.html
Muir, Bonnie M. “Trust between Humans and Machines, and the Design of Decision Aids.” International Journal of Man-machine Studies 27, no. 5–6 (1987): 527–539.
Replika. Accessed August 5, 2024. https://replika.com
Rheu, Minjin, Ji Youn Shin, Wei Peng, and Jina Huh-Yoo. “Systematic Review: Trust-Building Factors and Implications for Conversational Agent Design.” International Journal of Human–Computer Interaction 37, no. 1 (2021): 81–96.
Ryan, Mark. “In AI We Trust: Ethics, Artificial Intelligence, and Reliability.” Science and Engineering Ethics 26, no. 5 (2020): 2749–2767.
Silva, J. (2022). “Beneath the Hype: Self-Ethnography to Explore the Human Possibilities within NFT Technology.” Ethnographic Praxis in Industry Conference Proceedings (2022), 137–137. https://doi.org/10.1111/epic.12110
Upadhyaya, Nitish, and Matteo M. Galizzi. “In Bot We Trust? Personality Traits and Reciprocity in Human-Bot Trust Games.” Frontiers in Behavioral Economics 2 (2023): 1164259. https://doi.org/10.3389/frbhe.2023.1164259
Verma, P. (2023). “They Fell in Love with AI Bots: A Software Update Broke Their Hearts.” Washington Post, March 30, 2023, https://www.washingtonpost.com/technology/2023/03/30/replika-ai-chatbot-update
Vinkhuyzen, Erik, and Melissa Cefkin (2016). Developing Socially Acceptable Autonomous Vehicles. Ethnographic Praxis in Industry Conference Proceedings (2016), 522–534. https://doi.org/10.1111/1559-8918.2016.01108
Weitz, Katharina, Dominik Schiller, Ruben Schlagowski, Tobias Huber, and Elisabeth André. ““Do You Trust Me?’ Increasing User-Trust by Integrating Virtual Agents in Explainable AI Interaction Design.” Proceedings of the 19th ACM International Conference on Intelligent Virtual Agents (2019), pp. 7–9.
Whitham, Monica M. “Generalized Generosity: How the Norm of Generalized Reciprocity Bridges Collective Forms of Social Exchange.” American Sociological Review 86, no. 3 (2021): 503–531.
Zak, Paul J., and Stephen Knack. “Trust and Growth.” The Economic Journal 111, no. 470 (2001): 295–321.
Zhou, Michelle X., Gloria Mark, Jingyi Li, and Huahai Yang. “Trusting Virtual Agents: The Effect of Personality.” ACM Transactions on Interactive Intelligent Systems 9, no. 2–3 (2019): 1–36.