As a researcher, I often reflect on my practice and the impact of what I do. I think about how tech is evolving and I consider how I can take part in boosting the benefits and lessening the risks. As a responsible researcher, I want to ensure that my methods and results are honest, purposeful and ever-evolving and that I can let go of beliefs that might no longer serve me. There are myths and misconceptions around the objectivity of quantitative research and the neutrality of tech and the two are linked, as we’ll see. At best they lead organizations to embrace half-truths, and at worst they result in discrimination. By embracing our humanity and using our own subjectivity to critically examine the ways we research, we can prioritize our work in a way that aligns with ethical values and brings humans to the center.
Myth #1: Tech is Neutral
When I started working in tech as a qualitative researcher, I felt intimidated by the quantitative approach that dominated that space. Quantitative information is often used as the only source to help business leaders make decisions, while qualitative insights often receive pushback. Yet, I had the intuition that understanding the depth of human experiences was far more essential than my technical colleagues suggested.
When I started, with my background in sociolinguistics, the corporate world of tech was unfamiliar to me. I wasn’t sure to what extent the industry would want to understand humans. Would I be empowered to represent people’s best interests? To what extent could my research be grounded in the scientific approach? I needed to understand technology and its environment better.
In general, humans are not at the center of the tech industry. However, I was lucky. My role was to bring humans and their needs into the mix. And I was grateful for the opportunity to highlight the good and the bad in the development of our products.
I soon realized that there is a widespread misconception that technology is morally and politically neutral. A common argument is that technology isn’t good or bad by nature, but it’s how someone uses it that gives it its value. This perspective suggests that technology is a tool, and people are responsible for using it ethically.
Yet, those working in tech are humans who shape technology both individually and collectively. The decisions made by those building technology affect the lives of everyone. A product team might decide that videos won’t show captions. This would have adverse effects of varying intensity on people – from those with poor loudspeakers or those who learn better through reading to those with severe hearing impairments.
Decision-makers in our organizations make choices based on the information available to them and the information they choose to trust. In the process of making decisions, we evaluate a limited number of alternatives. This process varies from rational to irrational, and can be based on explicit or implicit knowledge and beliefs. Knowledge has many gaps and we cannot possibly access all the data or give the available data equal priority. What we and our organizations choose to emphasize in research, design, and product development reflects the values and priorities of everyone involved in the process.
As a researcher, I am one link in the chain. Therefore, choosing a research position and methodology in a specific context is an ethical act.
Myth #2: The quantitative approach is objective
Researchers have been debating neutrality/bias and objectivity/subjectivity for almost as long as there has been research. We sometimes feel we need to fight to have our insights accepted and to prove their validity, especially if they are based on qualitative methods. A common topic of discussion amongst ourselves is how we can demonstrate the value of qualitative research in a world where everyone is bombarded with facts and figures all day.
Since I work in the industry, I frequently come up against the common assumption that quantitative data is inherently unbiased. Even if some people can think critically in conversations at the coffee machine, they soon stop questioning their own practice once they’re back at their desk.
Quantitative research approaches fall within the scope of the positivist tradition, which stems from the natural sciences. In this perspective, researchers are seen as objective analysts who distance themselves from personal values when conducting studies. Reality is deemed external to the observer. The world is perceived as made of “observable elements and events that interact in an observable, determined and regular manner.1”
AI is Biased
Further, today we have access to an unprecedented volume of data. The myth that “bigger numbers are always more believable 2 ” leads to the assumption that bigger is better. This, along with the availability of Big Data, has led to various issues, including how we’ve built algorithms. For instance, over the years, AI bias-related issues have been documented as giving rise to discrimination. This raises the question: To what extent are biases taken into account when data is collected and models are created? Unfortunately, rigorous testing of industry AI algorithms indicates that more often than not, AI reflects social dynamics and cultural norms. These studies uncovered significant stereotypical bias by gender, race, profession, and religion. For instance, Amazon found out in 2018 that an experimental version of its recruiting platform learned to favor male applicants based on historical data and current employee profiles. The technology prioritized candidates who were using words more commonly found on male engineers’ resumes. Amazon turned it off and pursued its continual effort to develop a toolkit to better detect biases in AI.3
Since the very purpose of machine learning algorithms is to categorize, classify and separate, discrimination is central to AI and its ever-growing application. An expert who deals with the potential fundamental rights challenges of AI and interviewed by the European Agency for Fundamental Rights states, “Making differences is not a bad thing as such. When deciding to grant a loan, credit history can be used to differentiate between individuals, but not on the basis of protected attributes, such as gender or religion. However, many personal attributes or life experiences are often strongly correlated with protected attributes. The credit history might be systematically different for men and women due to differences in earnings and job histories. 4 ” Therefore, building AI and using it to make decisions comes with a level of responsibility towards other humans. Because of this, awareness is vital. Companies need to be cognizant of the value systems and beliefs that permeate the development of their products. Only then can they take action to mitigate the risk of harmful consequences generated by their technology.
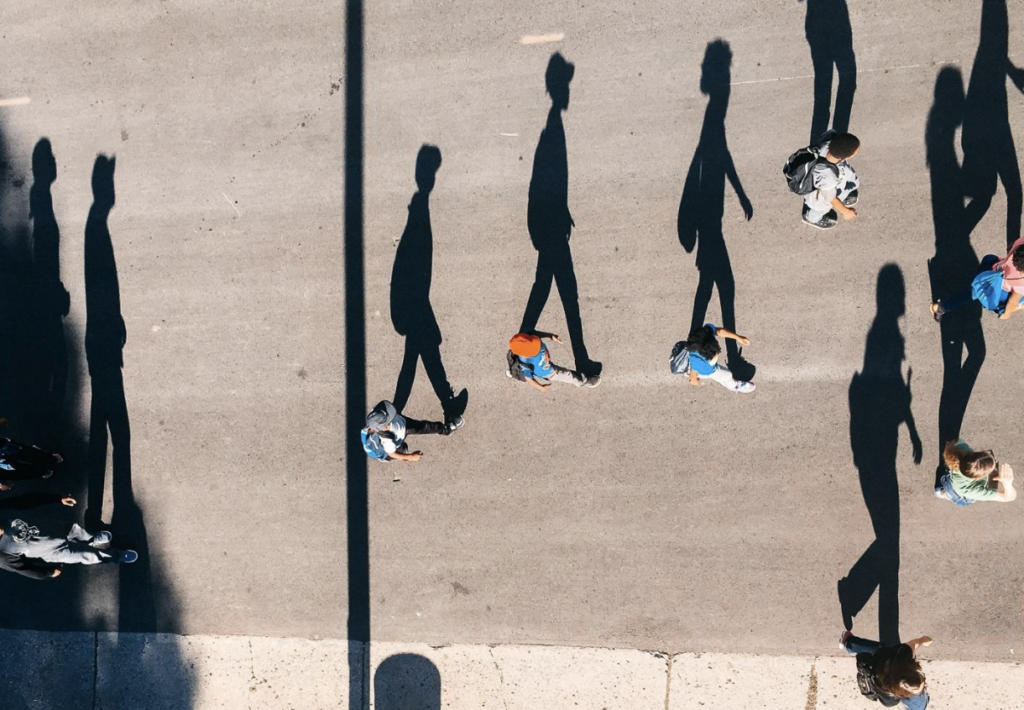
Biased input leads to biased output
Taking action includes examining data sets and their uses. Discrimination in AI decision-making can come from different sources, such as fragmented data sets (e.g. underreported crimes), errors in data sets (e.g. incorrect inputs), human implicit bias (while collecting and analyzing data), methodology (e.g. lack of internal validation, error rates, over-generalization). So, if predictive technologies are used to make decisions with no consideration for the above, they will reproduce existing discriminatory practices. In other words, algorithms trained on biased data will, without intervention, produce biased outcomes. In 2020, the Oxford Internet Institute’s Professor Sandra Wachter developed a bias detection tool. She explains that very often the data that predictive technologies use to decide if someone will go to university or be hired is biased because the world is biased. She says, “What happens is that the bad decision-making of the past finds its way into the future, but very often that happens in a very unintuitive non-obvious way. So we developed a tool that lets you test and find out if your hiring algorithm is fair to everybody equally.” Now, to test biases, we need metrics that reflect our value systems, including the ethical norms of our society. As we can see, algorithmic bias isn’t just a technical problem. We’re dealing with a social problem for which we need social sciences. Sandra Wachter concludes, “I cannot think of a single application now that doesn’t come from the social sciences in machine learning.5“
Social sciences as an integral part of AI development
Acknowledging the need for social sciences for all AI applications is a significant step forward. In this regard, the qualitative researcher has a big role in making everyone (in the company) aware of biases in general and people’s own positionality in particular. Our discipline offers us robust tools to reflect and think critically, as well as to research together with (and not on!) the people impacted by these systems. To achieve it, we need to move away from the idea that researchers are objective analysts that can entirely put aside their personal values in conducting their studies.
Participants’ and researchers’ subjectivity at the heart of the process
In parallel to the dominant positivist approach, a seemingly less prevalent constructivist approach has gained traction in large tech companies over the last few years. This qualitative approach looks at reality and seeks knowledge differently. The researcher is not external to their object of study and is not looking for an unattainable neutrality. And knowledge is not the neutral discovery of an objective truth. Rather, it’s the interaction between the researcher and their field of study that builds knowledge. Reality is not fixed, unchangeable or neutral. Instead, reality is regarded as “subjective, pluralistic, and elastic.6”
In this view, we don’t predetermine dependent or independent variables (as would usually be the case in quantitative, positivist research). Rather we focus on exploring and “giving an account of how people make sense of a situation at a particular point in time.7” The interactive link between the researcher and the object of research enables the findings to be created as the investigation unfolds. In this way, we use specific qualitative methods that enable the unexpected to arise. For example, we don’t use hypotheses at the beginning of the study; we allow participants to drive the direction of the interview. We let what we haven’t thought about simply reveal itself, rather than verifying what we have already thought about.
We also recognize that human values are involved in research. Values refer to the moral principles and beliefs that we think are important. As a researcher I’m aware that I am an individual embedded within a particular society with my upbringing, my values and my viewpoints. Practically, from planning and data collection to the analysis and final report, the researcher’s voice is not hidden. On the contrary, it is explicitly acknowledged! We must accept and embrace our own subjectivity rather than try to eliminate it from the results. First, we attempt to prioritize the data over the researcher’s assumptions and existing knowledge. We then think about it critically. For example, a middle-aged female researcher with no disability is interviewing teenage girls with motor impairment. She might consider ways in which she is and is not armed to understand their perspectives.
In organizations where we don’t have the time to think about these questions, the underlying philosophy guiding research principles is often understated, unspoken, or even considered irrelevant. Sometimes researchers are unaware of their own positionality, that is how their identity influences their outlook on the world. The danger here is that without this questioning, they might ultimately follow what they believe is expected from them by the organization. Take automation as an example. Automation is often seen as a positive force for businesses, increasing revenue or reducing costs. Teams accept this perspective without much scrutiny. In this context, researchers might overlook examining the fundamental implications of automation itself. They may not realize that their view of automation is influenced by cultural, economical, political factors. Neglecting these perspectives, researchers may overly concentrate on the design of automation while missing the fact that automation may not meet users’ bigger goals.
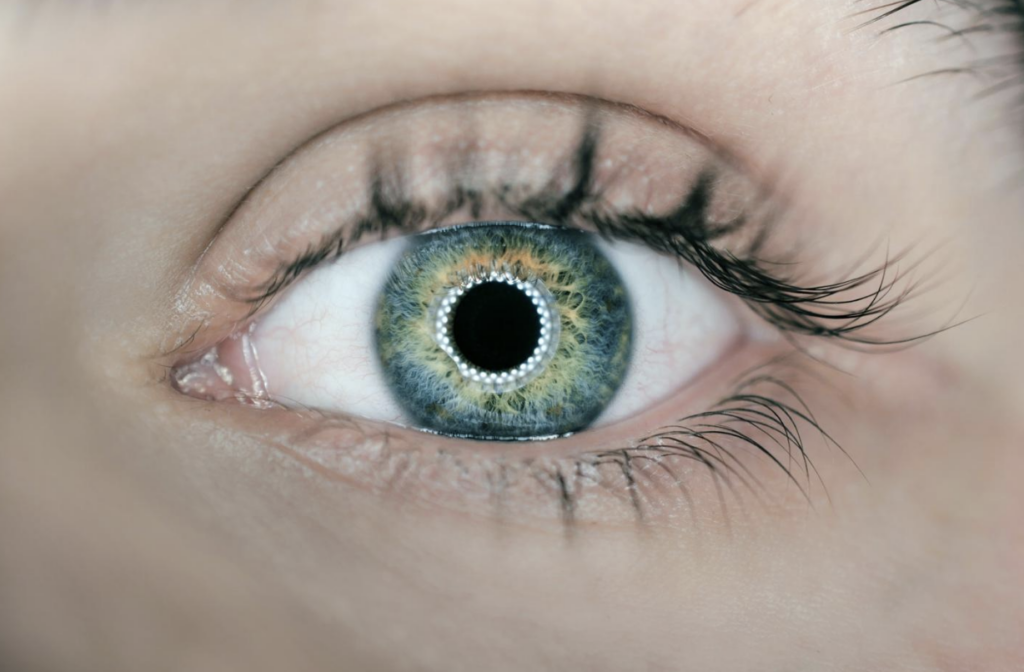
Conclusion
Researchers’ positions directly influence research methodologies and operations. Therefore, selecting a research design that accounts for positionality is vital.
- We must begin with awareness. If we are aware, we can make the effort to examine our feelings, reactions, and motives and how these influence our actions and thoughts during our research. We can consciously, ethically, and honestly choose our position of research. This concept helped me improve my own research practice. Recently I started journaling during research projects. This helps keep me become more aware of my values while investigating, interpreting data, and selecting insights to share.
- Secondly, there is a valuable knock-on effect. Our philosophical position reflects a particular worldview. This points us towards specific methods that uncover insights leading to decisions that impact people’s lives.
- Thirdly, it is important that we select the right qualitative methods to help us to get closer to all aspects of being human. This is particularly valuable when researching the realities of underrepresented groups of people.
Finally, as researchers, I suggest we question our practice by regularly reviewing and expressing our positionality, and choosing a paradigm and methodologies that align. Choosing a position, a framework and a methodology in a specific context is an ethical act.
Booking.com is an EPIC2023 sponsor. Sponsor support enables our annual conference and year-round programming that is developed by independent committees and invites diverse, critical perspectives.
DEFINITIONS
Algorithm: a sequence of commands for a computer to transform an input into an output.8
Artificial intelligence (AI): software (and possibly also hardware) systems designed by humans that, given a complex goal, act in the physical or digital dimension. To do this, they perceive their environment through data acquisition, interpret the collected structured or unstructured data, reason on the knowledge, or process the information, derived from this data. They then decide the best action(s) to take to achieve the given goal.9
Discrimination: where one person is treated less favorably than another is, has been or would be, treated in a comparable situation based on a perceived or real personal characteristic.10
REFERENCES
Bisman, Jayne E. , and Charmayne Highfield. 2012. “The Road Less Travelled: An Overview and Example of Constructivist Research in Accounting.” Australasian Accounting, Business and Finance Journal. 2012. https://ro.uow.edu.au/aabfj/vol6/iss5/2/.
Brinson, Sam. 2020. “Is Technology Neutral?” November 17, 2020. https://medium.com/understanding-us/is-technology-neutral-39d5b445b315.
Collins, Hilary J. 2010. Creative Research the Theory and Practice of Research for the Creative Industries. London Bloomsbury Visual Arts.
Dastin, Jeffrey. 2018. “Amazon Scraps Secret AI Recruiting Tool That Showed Bias against Women.” Reuters. October 11, 2018. https://www.reuters.com/article/us-amazon-com-jobs-automation-insight/amazon-scraps-secret-ai-recruiting-tool-that-showed-bias-against-women-idUSKCN1MK08G.
European Union Agency for Fundamental Rights. 2020. “Getting the Future Right – Artificial Intelligence and Fundamental Rights.” Publications Office of the European Union. https://fra.europa.eu/sites/default/files/fra_uploads/fra-2020-artificial-intelligence_en.pdf.
European Union Agency for Fundamental Rights. 2022. “Bias in Algorithms – Artificial Intelligence and Discrimination.” Publications Office of the European Union. https://fra.europa.eu/sites/default/files/fra_uploads/fra-2022-bias-in-algorithms_en.pdf.
High-Level Expert Group on Artificial Intelligence. 2019. “A Definition of AI: Main Capabilities and Scientific Disciplines.” European Commission.
Nadeem, Moin, Anna Bethke, and Siva Reddy. 2020. “StereoSet: Measuring Stereotypical Bias in Pretrained Language Models.” ArXiv (Cornell University), April. https://doi.org/10.48550/arxiv.2004.09456.
Stevens, Molly, and Lukas Vermeer. 2021. “Multiplication instead of Division.” May 2021. https://medium.com/booking-product/multiplication-instead-of-division-7b57d9d7800b.
University of Oxford’s Social Sciences Division. 2022. “The Bias Detection Tool Developed at the University of Oxford and Implemented by Amazon.” Www.youtube.com. March 16, 2022. https://www.youtube.com/watch?v=MNmR6068vQg&t=1s.
Photos
Photo 1 by Tom Barrett on Unsplash
Photo 2 by Arteum.ro on Unsplash
- Collins, H.J. (2010). Creative research the theory and practice of research for the creative industries. London Bloomsbury Visual Arts, p.38. ↩︎
- Stevens, Molly and Lukas Vermeer. 2021. Review of Multiplication instead of Division. May 2021. ↩︎
- Dastin, J. (2018). Amazon scraps secret AI recruiting tool that showed bias against women. [online] Reuters. ↩︎
- European Union Agency for Fundamental Rights. 2020. “Getting the Future Right – Artificial Intelligence and Fundamental Rights.” Publications Office of the European Union. ↩︎
- University of Oxford’s Social Sciences Division. 2022. “The Bias Detection Tool Developed at the University of Oxford and Implemented by Amazon.” ↩︎
- Bisman, Jayne E., and Charmayne Highfield. 2012. “The Road Less Travelled: An Overview and Example of Constructivist Research in Accounting.” Australasian Accounting, Business and Finance Journal. 2012. ↩︎
- idem. ↩︎
- European Union Agency for Fundamental Rights. 2022. “Bias in Algorithms – Artificial Intelligence and Discrimination.” Publications Office of the European Union. ↩︎
- High-Level Expert Group on Artificial Intelligence. 2019. “A Definition of AI: Main Capabilities and Scientific Disciplines.” European Commission. ↩︎
- European Union Agency for Fundamental Rights. 2020. “Getting the Future Right – Artificial Intelligence and Fundamental Rights.” Publications Office of the European Union. ↩︎
0 Comments